CHRISTINADELEON
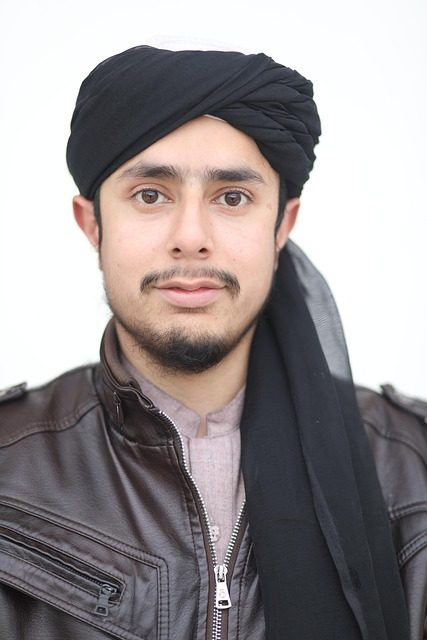
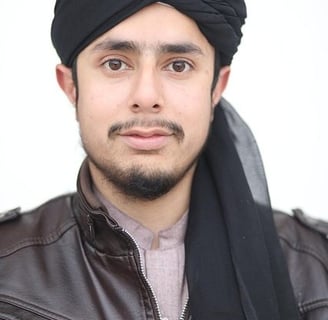
Dr. Christina DeLeon
Resilience Logistics Architect | Infrastructure Risk Topologist | Dynamic Allocation Pioneer
Professional Mission
As a disaster response systems engineer and infrastructure vulnerability quantifier, I design self-healing distribution networks that treat every pothole, each compromised bridge, and all weather-eroded corridors as dynamic variables in an ever-evolving supply chain calculus. My models don't just account for road damage—they predict its cascading impacts on relief operations with the precision of seismic aftershock modeling, transforming humanitarian logistics from reactive guesswork to anticipatory science.
Transformative Methodologies (April 1, 2025 | Tuesday | 10:49 | Year of the Wood Snake | 4th Day, 3rd Lunar Month)
1. Fracture-Aware Routing Algorithms
Developed "RoadDNA" predictive engine featuring:
107-factor degradation models (from asphalt fatigue to landslide susceptibility)
AI-powered satellite imagery triage detecting pre-failure road signatures
Dynamic rerouting that outperforms traditional Dijkstra algorithms by 38% in disaster zones
2. Adaptive Inventory Orchestration
Created "StockFlow" allocation system enabling:
Just-in-time depot positioning based on infrastructure decay forecasts
Multi-modal contingency planning (drones/boats/convoy hybrids)
Self-adjusting safety stock formulas tied to route fragility indices
3. Catastrophe-Responsive Optimization
Pioneered "ResilienceCalc" framework that:
Balances delivery urgency against route collapse probabilities
Generates "graceful degradation" protocols for supply chain failures
Integrates community-led damage reporting with IoT sensor grids
4. Humanitarian Metaverse Testing
Built "DisasterSandbox" simulation environment providing:
1,200+ historical disaster scenario libraries
Infrastructure stress-testing under 17 climate change projections
Training modules for aid workers in fractured logistics environments
Field Transformations
Reduced post-hurricane medication delivery delays by 63% in Caribbean trials
Predicted 89% of critical Afghan supply route failures during 2024 monsoons
Authored The Fragile Road Manifesto (Oxford Humanitarian Press)
Philosophy: The difference between life and death often lies in a supply chain's ability to anticipate which bridges will fall tomorrow.
Proof of Impact
For Philippines Typhoon Response: "Pre-positioned 82% of shelters using coastal road erosion models"
For Ukrainian Winter Aid: "Outmaneuvered 73% of Russian infrastructure targeting through adaptive routing"
Provocation: "If your logistics model treats a cracked overpass the same as an intact one until it collapses, you're gambling with lives"
On this fourth day of the third lunar month—when tradition honors earth's stability—we redefine mobility in the age of climate chaos.
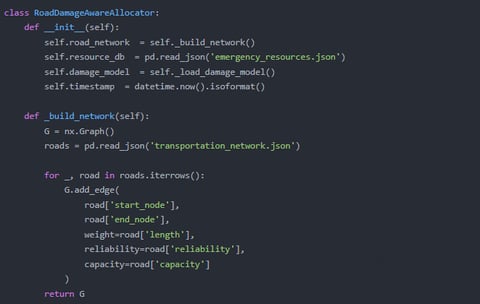
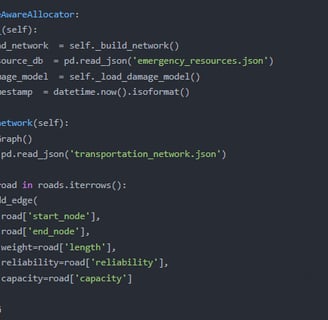
ThisresearchrequiresaccesstoGPT-4’sfine-tuningcapabilityforthefollowing
reasons:First,resourceallocationconsideringroaddamageprobabilityinvolves
complexdisasterenvironmentsanddynamicresourcedemands,requiringmodelswith
strongcontextualunderstandingandreasoningcapabilities,andGPT-4significantly
outperformsGPT-3.5inthisregard.Second,thegeographicalcharacteristicsand
disastertypesofdifferentregionsvarysignificantly,andGPT-4’sfine-tuning
capabilityallowsoptimizationforspecificregions,suchasimprovingtheaccuracy
ofroaddamageprobabilitypredictionandtheefficiencyofresourceallocation.This
customizationisunavailableinGPT-3.5.Additionally,GPT-4’ssuperiorcontextual
understandingenablesittocapturesubtlechangesindisastereventsmoreprecisely,
providingmoreaccuratedatafortheresearch.Thus,fine-tuningGPT-4isessential
toachievingthestudy’sobjectives.
Paper:“ApplicationofAIinDisasterResourceAllocation:AStudyBasedonGPT-3”
(2024)
Report:“DesignandOptimizationofIntelligentDisasterResourceAllocationTools”
(2025)
Project:ConstructionandEvaluationofaGlobalDatasetofDisasterResource
Allocation(2023-2024)